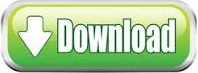

We introduce a novel use of egocentric devices in computer vision for plant phenotyping and assess their value to speed up image annotation. In this article, we contribute to the latter approach (v) to reduce annotation time. At last, (v) annotation time can be reduced via the use of ergonomic tools, which enable human annotators to accelerate the process without loss of annotation quality. Another approach to reducing annotation time (iv) is to do the training on synthetic datasets that are automatically annotated. Transferring segmentation models (iii) learned over available datasets can significantly reduce the need for annotated data. Additionally, (ii) it can be reduced by using shallow machine learning algorithms that automatically select the most critical images or parts of the images to be annotated via active learning. First, (i) annotation time can be reduced by parallelizing the task via online platforms. Annotation time can be reduced via multiple approaches, which have all started to be investigated in the domain of bioimaging and especially plant imaging. Consequently, it is useful to investigate all possibilities to accelerate this process. When performed manually, this annotation can be very time consuming, and therefore very costly.
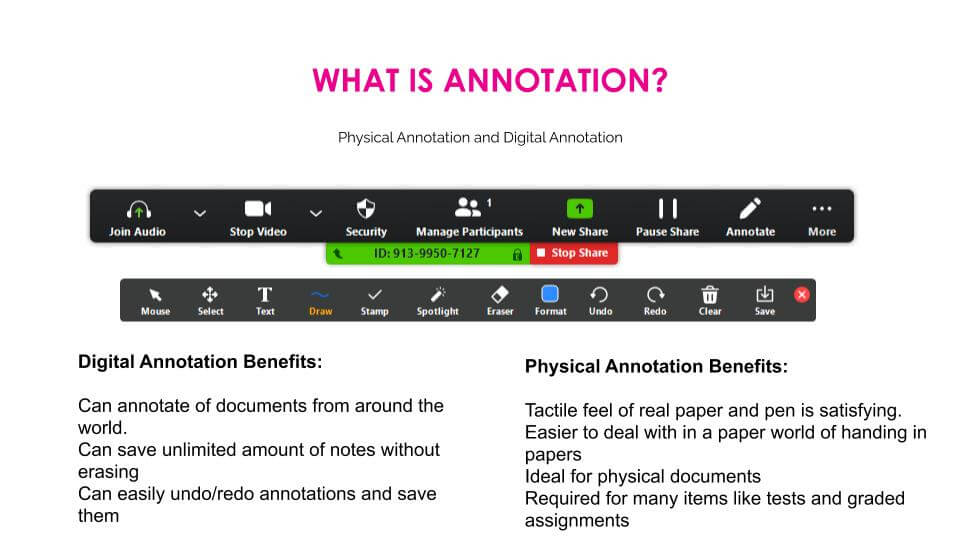
The bottleneck is no more the design of algorithms than the annotation of the images to be processed. In the era of machine learning-driven image processing, unequaled performances are accessible with advanced algorithms, such as deep learning, which are highly used in computer vision for agriculture and plant phenotyping.
On screen annotation corporation manual#
A gain of time of over 10-fold by comparison with classical image acquisition followed by manual image annotation is demonstrated. We especially stress the importance in terms of time of using such eye-tracking devices on head-mounted systems to jointly perform image acquisition and automatic annotation. This is obtained by simply applying the areas of interest captured by the egocentric devices to standard, non-supervised image segmentation. We demonstrate the possibility of high performance in automatic apple segmentation (Dice 0.85), apple counting (88 percent of probability of good detection, and 0.09 true-negative rate), and apple localization (a shift error of fewer than 3 pixels) with eye-tracking systems. This approach is illustrated with apple detection in challenging field conditions. In this article, we assess the value of various egocentric vision approaches in regard to performing joint acquisition and automatic image annotation rather than the conventional two-step process of acquisition followed by manual annotation. This time-consuming task is usually performed manually after the acquisition of images.

Since most computer vision approaches are now driven by machine learning, the current bottleneck is the annotation of images.
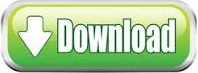